
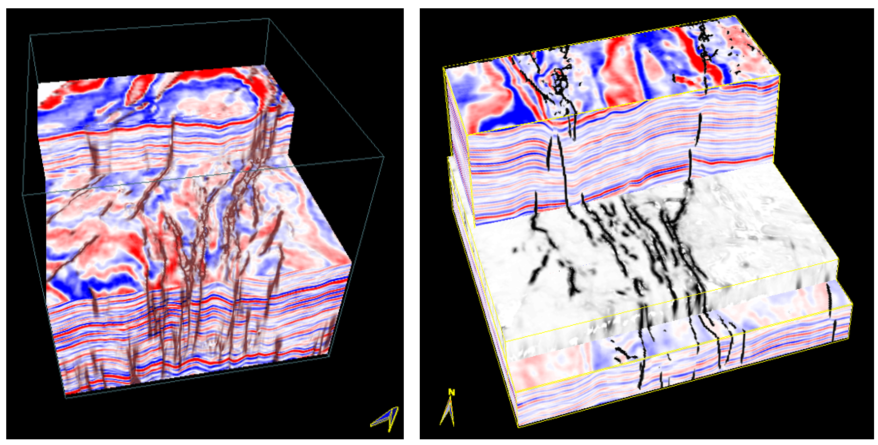
Predictive painting generates structure-preserving predictions of seismic events from neighboring traces, whereas similarity-mean filtering reduces several predictions to optimal output values. In this paper, we combine predictive painting with nonlinear similarity-mean filtering to define a structure-enhancing filter. (2009) introduced time-dependent smooth weights to design a similarity-mean stack, which is a nonstationary, nonlinear operator. Rashed (2008) proposed “smart stacking,” which is based on optimizing seismic amplitudes of the stacked signal by excluding harmful samples from the stack and applying more weight to the center of the sample population. Robinson (1970) proposed a signal-to- noise-ratio-based weighted stack to further minimize noise. Alterative stacking methods were therefore proposed, along with improvement in optimizing weights of seismic traces. Conventional stacking is a simple and effective method of de-noising, but it is optimal only when noise has a Gaussian distribution (Mayne, 1962). Mean filter (stacking) plays an important role in improving signal-to-noise ratio in seismic data processing (Yilmaz, 2001). The predictive painting method, introduced recently by Fomel (2008), provides yet another alternative, with superior computational performance. Stark (2003) presented an alternative approach involving instantaneous phase unwrapping. The idea of using local shifts for automatic flattening was introduced by Bienati and Spagnolini (2001) and Lomask et al.
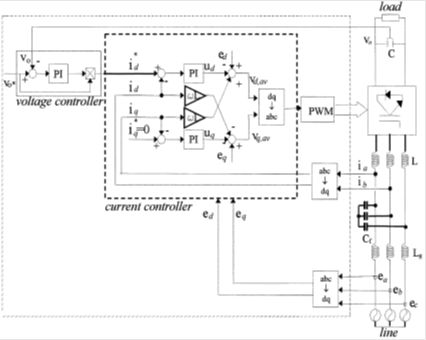
Seismic flattening (stratal slicing) can improve the interpreter’s ability to explain and quantify the structural architecture of sedimentary layers (Zeng et al., 1998). The main challenge in designing effective structure-enhancing filters is controlling the balance between noise attenuation and signal preservation. Random noise attenuation and structure protection are always ambivalent problems in seismic data processing. (2008) introduced a frequency-dependent, structurally conformable filter. Traonmilin and Herrmann (2008) used a mix of finite-impulse-response (FIR) and infinite-impulse-response (IIR) filters, followed by f-x filtering, to perform structure-preserving seismic processing. Fomel and Guitton (2006) suggested the method of plane-wave construction. Fehmers and Höcker (2003) applied structure-oriented filtering based on anisotropic diffusion. (2006) applied nonlinear filters, such as median, trimmed mean, and adaptive Gaussian, over planar surfaces parallel to the dip.

A number of approaches have been proposed to preserve and enhance structural information. Numerical tests using synthetic and field data confirm the effectiveness of proposed structure-enhancing filtering.Įxtracting structural information is the most important goal of seismic interpretation. Parameters of the nonlinear filter allow us to control the balance between eliminating random noise and protecting structural information. We apply a nonlinear similarity-mean filter to select the best samples from different predictions. We use predictive flattening to form a structural prediction of seismic traces from neighboring traces. The method is based on combining predictive flattening with similarity-mean filtering. We present a novel filtering method, which aims at reducing random noise while protecting seismic structures. INTRODUCTION SUMMARY Attenuation of random noise and enhancement of structural continuity can significantly improve the quality of seismic interpretation.
